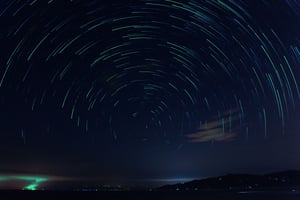
Getting Value from AI? The 2024 State of the Data Center Report Could Help
Investments in AI are at an all-time high. Is the money well spent? It depends – are you taking a disciplined approach to AI? Insights from the 2024 State of the Data Center Report by Foundry and CoreSite can help you make “smart AI” decisions.
The AI Adoption Trend
Business leaders are rushing to formalize, fund and launch AI initiatives. Individual use of AI is also rising quickly via tools such as ChatGPT and Anthropic.
According to a May 2024 McKinsey survey report on AI, 65% of respondents say their organizations are regularly using GenAI, nearly double the percentage from a survey 10 months prior. The survey finds that global AI adoption has jumped to 75% compared to 2023, when AI adoption did not reach 66% in any region.
Almost all (97%) of business owners believe ChatGPT will help their business, and nearly
two-thirds (64%) believe AI will improve customer relationships.1
As you can see in the McKinsey charts, marketing and sales functions are the top users of AI, followed by product and/or service development. Within functions, AI use cases include content development, chatbots and assistants, personalized marketing and data management.
Compare a Vue.ai study to the McKinsey findings. Vue.ai focuses on how 10 industries use AI. The top use cases are:
- Data cataloging and management – Organizations spend 80% of their time looking for data and only 20% of the time utilizing it. AI is reducing data management efforts and search times.
- Customer experience management – AI can help enrich customer profiles and seamlessly apply real-time insights across the conversion funnel.
- Workflow automation – By integrating AI into their workflows, teams can streamline processes, uncover inefficiencies and deliver quicker conversion down the value chain.
- Personalization – With personalization, companies can deliver the most relevant piece of content to every user at the right time.
- Demand forecasting and optimization – AI-powered forecasting can reduce errors by 30-50% in supply chain networks.
- Churn prevention – AI inference models enable identifying the precise indicators of churn.
Other common use cases include AIOps; virtual assistants for customers, employees and data; software code agents; predictive maintenance; and cyber threat detection and response. Google provides 101 examples of companies and how they’re using AI.
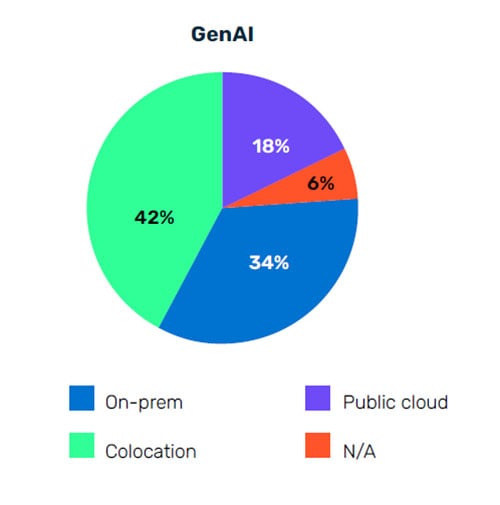
Why AI Workloads Are Moving into Colocation
The rise of AI use begs the question: Where are organizations hosting GenAI workloads and why? According to the 2024 State of the Data Center report, 42% are in colocation, 34% in on-premises data centers and 18% in public cloud.
Lower data center costs are a primary reason for moving AI workloads into colocation. However, the 300+ IT leaders surveyed for the State of the Data Center report say that other drivers are:
From on-premises environments to colocation:
- Security
- Reliability
- Performance/speed
From public cloud to colocation:
- Security and compliance
- Scalability
- Simplicity
For GenAI and machine learning workloads specifically, the report identifies the top three reasons why they’re placed in a colocation data center.
- Cloud interconnection
- Security
- Expertise in enabling AI applications
In fact, cloud interconnection is the No. 1 reason for all workloads to move to colocation. Second- and third-place reasons vary by workload type. IT leaders recognize that a colocation data center provider is the preferred option to address challenges such as complexity, interconnection requirements, need for flexibility, and risk to a critical business asset – data.
Are AI Investments Paying Off?
The Stanford AI Index Report finds that “Training state-of-the-art AI models has become extraordinarily expensive. OpenAI’s GPT-4 cost $78 million to train, Google’s Gemini Ultra $191 million.”2 According to the study, funding for GenAI increased 8x, totaling $25.2 billion in 2023.
Either the investments are paying off or decision makers are banking on AI-based innovation to produce positive results. It’s likely that both are in play. The Stanford study respondents say that AI makes workers more productive and leads to higher quality work while noting that other studies caution that using AI without proper oversight can lead to diminished performance.3 While anecdotal information is interesting, tangible gains will convince stakeholders that investments are worthwhile.
ServiceNow’s Enterprise AI Maturity Index study finds that 74% of AI pacesetters are seeing a positive return on investment, and one in three is hitting returns of 15% or more. Other research cites an average of 66% improvement in user performance from the use of GenAI tools for customer support, writing business documents and programming.4 Another example – software developers can complete coding tasks up to 2x faster with GenAI.5
The Reality of AI Ups and Downs
Presumably, use cases are scrutinized closely before they are rolled out. Yet AI integration isn’t all smooth sailing. Chatbots and other virtual assistants can provide incorrect information. AI-generated content that doesn’t meet an organization’s editorial standards could be published anyway. Employee recruiting software can discriminate against certain applicants. Stealth use or initiatives that bypass IT/security increase risk related to cyber threats, compliance, privacy and so on.
Sometimes, less-than-optimal outcomes result from omissions early in the AI lifecycle:
- Lack of alignment between AI strategy and business directives and guardrails.
- Workers aren’t trained in the use of AI tools or data quality and collection. Data quality is everything in training AI models. Relevant data may be scattered across an organization, and it can be overlooked when functions aren’t collaborating, or data inventories are outdated. IDC reminds us that 82% of organizations report siloed data.6
- AI initiatives aren’t subject to rigorous risk assessment or risk management policies.
Not all risk can be avoided, but following best practices gives you a better chance of minimizing errors, supporting compliance and preventing costly missteps.
Lean into Best Practices to Keep AI Decisions on Track
Another CoreSite blog discusses the basics of AI – types, value and applications – and it’s a good lead into best practices, including these five tips:
-
Develop your organization’s AI policy. It should address topics such regulations, accountability, transparency and data privacy/protection. Educate employees, partners and suppliers about the policy and how you expect to enforce it. ISACA provides useful guidance.
-
Create an AI strategy, including rules, guardrails and a formula for evaluating use cases and proofs of concept. The Balanced Scorecard Institute offers tips.
-
Choose appropriate system architecture. Organizations are increasingly turning to colocation because in-house power, cooling and interconnection aren’t fiscally sustainable or resources are inadequate:
-
Excessive cost to build and scale – intense computing requires leading-edge hardware, power and cooling
-
Inability to attract and hire people with AI skills
-
Time-consuming compliance audits and reporting
-
Lack of essential functionality such as cloud interconnection
-
-
Follow a FinOps framework to bring together technologists, the finance team and business leaders. This collaborative approach focuses on maximizing cloud investments. FinOps can reduce cloud costs.
-
Prioritize governance, along with security, privacy and compliance. Define the rules and standards that guide the use of AI tools in ethical, safe and compliant ways.
Get More Bang for Your AI Bucks with Colocation
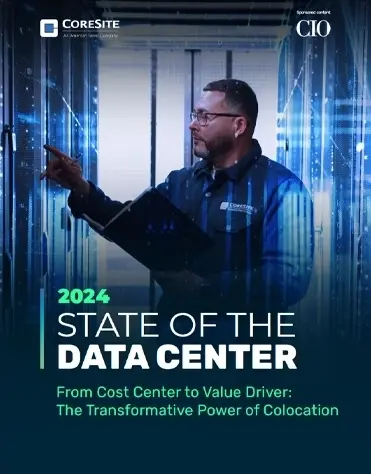
Download today
According to the 2024 State of the Data Center Report, three out of four respondents say that AI is an accelerant to colocation adoption. They’re considering moving AI-related workloads, including GenAI applications, chatbots, predictive analytics and augmented AI applications, to a colocation data center.
CoreSite has developed a series of persona-based AI adoption journeys. You can go along. To learn about the challenges faced by IT leaders and AI users in Biopharma, Retail, IT Engineering and FinTech while implementing AI – and how they conquered those challenges – download the 2024 State of the Data Center report.
As a certified NVIDIA DGX-ready data center partner, CoreSite hosts AI, machine learning (ML) and other high-density applications that require scalability and high performance. Our infrastructure enables you to store massive amounts of data, power AI workloads (they require more than 30 kW per rack), provide various cooling solutions and access clouds directly.
References
-
Forbes, How Businesses Are Using Artificial Intelligence in 2024
-
Stanford University, The AI Index Report, Measuring Trends in AI, 2024
-
Ibid
-
Nielsen Norman Group, AI Improves Employee Productivity by 66%, July 16, 2023
-
McKinsey, Unleashing Developer Productivity with Generative AI, June 27, 2023
-
IDC blog, Planning for Success with Generative AI, August 25, 2023