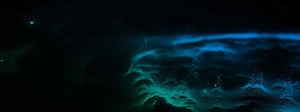
To Deploy Tomorrow’s AI Workflows, Starting Today Is Already Too Late
We find ourselves in an ecosystem where everyone wants to simultaneously leverage new, power-intensive technologies—and even with the feverish pace of data center construction, there simply isn’t enough space for everyone who wants it. In the wake of 2023’s “Year of AI,” we’re now living in what is arguably a fourth industrial revolution: The new technologies that the world came to understand last year are now being deployed at unprecedented pace and scale, and not just in manufacturing. For nearly a decade, the percentage of organizations utilizing AI in one or more of their critical business functions hovered in the 50s; since last year, that number has risen to nearly 75%, with GenAI use, specifically, doubling over that time period.
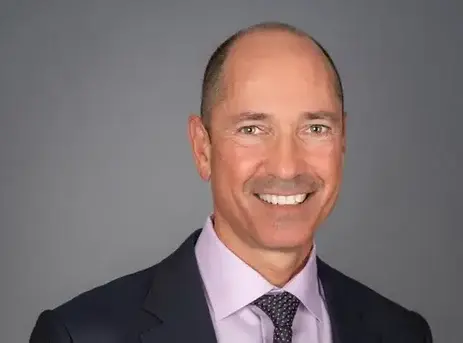
Organizations are racing to deploy GenAI tools—but the process of bringing these capabilities to bear doesn’t occur in a vacuum. The challenges of rapid and expansive AI deployment have manifested as a nascent technology arms race, with household-name tech companies battling for “chip supremacy” of their graphical processing units (GPUs) and, in the process, market capitalization. For enterprise leaders, the need to obtain enough of the most powerful chips, as well as the infrastructure to run them, has become imperative. But with demand far outstripping supply of energy, raw materials and data center capacity, the result is a strained technology ecosystem, with companies looking for information that will enable them to plan with some certainty when they could integrate AI workflows.
Confronting A Shortage Of Power
We are in a period of particularly robust demand for data center capacity—and the single biggest driver is GenAI. The reason for this is that GenAI programs utilize GPU chips (usually the ones created by market-leader NVIDIA) as opposed to less power-expending CPUs, like the kind that run processes in your laptop. The training models that these AI tools require consume some five to 10 times more power than their predecessors, and with the incredible proliferation of GPU-dependent AI platforms, power companies are having a hard time satisfying the demand. The power grid in places like Northern Virginia or Silicon Valley, hotbeds of data center growth for decades, can no longer support the current rate of growth.
The result has been a shift to more geographically distributed data center architecture, where the hyperscalers—that is, companies that build and/or occupy very large data centers (such as Microsoft, Google, Amazon, or Meta)—are suddenly developing data center campuses in places that have not previously been Tier 1 cloud regions, such as Atlanta, Reno, and Charlotte. These hyperscalers are looking to find places with enough land and power available to effectively “feed” the AI beasts for years to come. The result is a far more distributed cloud and AI architecture; the constraints that the power and utilities are placing on the ability to sustain growth in traditional locations is causing a shift in where clouds “live.”
A Search For New Hosts
The cloud is, in a sense, old news—it has been “the” utility for the past 15 years. The AI revolution is serving as an accelerant to a host of other demand-drivers within cloud platforms that already were there. Now, they are effectively turbocharged. Many of the enterprises we cater to are dependent on the ability to connect to the cloud, whereupon they host and execute a variety of workloads that are a mainstay of their operations. Now, when those workloads become AI workloads, the amount of data transfer and compute required is much higher.
The unprecedented explosion of these more power-intensive workloads impacts the entire technology ecosystem. It is not just the large learning models that must be deployed, but also myriad smaller, custom models need to be trained and then you need to support the model inferencing itself, and the power and materials (more on those in a moment) that go along with establishing this AI infrastructure. Many companies that previously depended on their own cloud are having a hard time deploying infrastructure in their data centers that can even remotely support the amount of power necessary for this new wave of GPU chips. This has led to increased outsourcing. Companies that previously relied on on-premises data centers are now looking to colocate and, given the aforementioned supply shortage, some enterprises and digital platforms are pre-leasing data center space for years in advance. So, as I said at the top of this post, we find ourselves in an ecosystem where everyone wants to simultaneously leverage these new, power-intensive technologies—and even with the feverish pace of data center construction, there simply isn’t enough space for everyone who wants it.
Bottlenecks In The Supply Chain
The shortages we’re seeing occur on two main levels: infrastructure and equipment. At a basic level, we need land, water, power, and fiber to construct data center campuses where real estate is in high demand. Utility companies are increasingly strained, which presents a major obstacle for both data center providers and enterprises trying to run these power-intensive processes.
Another obstacle is obtaining materials and equipment. For the equipment needed to build-out data centers, particularly generators, switchgears and transformers, there is now tremendous demand due to very elongated production cycles. For example, there is a wait list of around two years for generators. For context, it used to be around three months. So, companies must plan two years ahead if they need generator capacity to support load growth related to GPU proliferation. Some companies are buying five or six years in advance, or more, which puts other companies in the position of also having to plan much further in advance to support their own infrastructure.
Once a data center is built, the customers’ equipment that goes into it, such as NVIDIA servers, can also be very hard to buy. Some server and chip shortages are due to backlogs that still exist from the pandemic, but most are a result of the surge in demand for this advanced equipment, as more and more platforms are dependent upon AI, and thus GPUs. The result is supply chain stress at every juncture, with hyper-scaled companies remaining the only ones with the ability to buy at the capacity needed, even before a data center has been built.
The Imperative For Future Planning
The reality is that, despite any skepticism or temporary market dips, AI is a relentless juggernaut. For enterprise leaders, the question of how best to deploy new AI-driven services or use AI to develop products isn’t simply one of innovation, but one that includes serious consideration of resource availability. The scramble for data center capacity, bottlenecks in supply chains and the constraints on energy and other utilities all mean that they can no longer expect to immediately implement their AI projects. Rather, in most cases, they will contend with years-long wait times before the necessary resources are available.
Consequently, organizational leaders must think more strategically and further in advance than ever before. They need to consider what different ways they’ll be interacting with their customers in the future. What processes will be integral to their operations? What data will they be utilizing? What AI workloads will they be implementing? And, crucially, what infrastructure will they need to support these workflows? Will their applications require a level of power and cooling that can be delivered in-house? Or, will they be dependent on third-party data center capacity? And of course, as the prices of materials, energy and space all increase, what will be the associated costs?
In essence, the technology arms race is a contest of forethought: Only by planning ahead can companies truly manifest their own digital transformation.